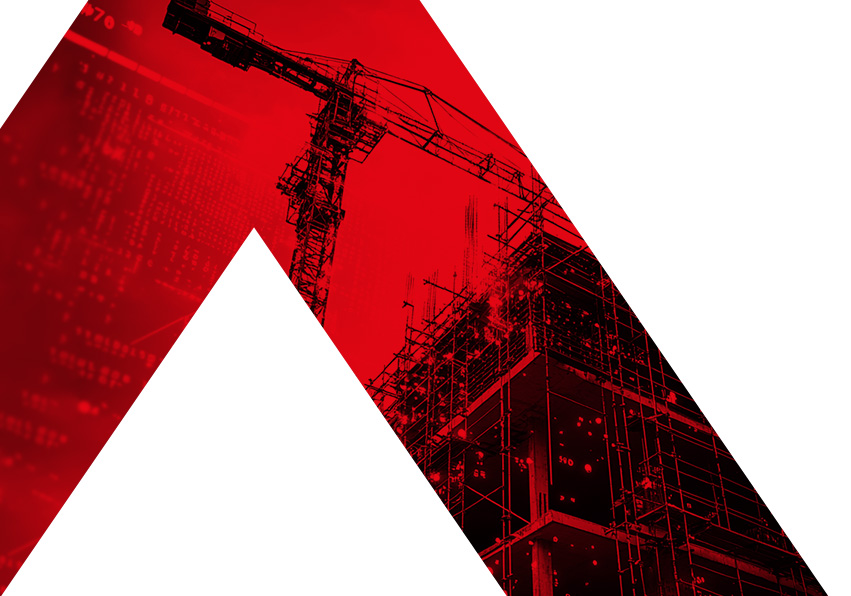
Artificial Intelligence.
Real Abilities.
Physical AI is an AI deployed into real-world tasks via robots
Unlike digital AI, Physical AI requires data from dynamic, real-world environments. This makes clean, information-rich data the most important aspect of Physical AI development.
Before an AI model can be uploaded to a real-life robot body, it needs to be improved by running millions of simulations in a digital environment.
However, the scarcity of high-quality physical data creates significant challenges that slow down innovation and real-world implementation.
Building datasets & training grounds for Physical AI
Real abilities for Physical AI built from Datasets, World Models & deployment-reinforced learning.
AIRA built a scalable data acquisition process that collects model-accurate, context-rich data from physical projects in the Built Environment.
AIRA builds time & space-accurate World Models and Simulation Training Grounds for Physical AI based on real-life data from unstructured jobs, most relevant for automation.
Data acquisition & transformation
Building realistic World Models and training Large Behaviour Models (LBM) needed for Physical AI will require vast amounts of high fidelity temporal data from 1,000s of productive environments.
To achieve this, AIRA developed value-add services underpinning both the data acquisition activity and adoption of Data Scouts, instantly unlocking the value of data.
Current AI models lack the awareness and understanding of the physical world.
Current AI models are limited to impacts in the digital landscape
Training AI to provide a useful contribution to physical actions through robots requires up to 100,000,000s of hours of real-life deployment. Every Autonomous Vehicle driver is, in effect, a coach of a complex AI system which collects inputs affecting the car and outputs (driver’s reactions), learning more with every ride. In other areas ‘ghosting’ (Guided Reinforcement Learning) of drone operators, enables data to be used to teach AI about flight. As much as this process can be effective, replicating this in other environments has proven extremely hard.
And yet, self-driving cars, after a decade of development and $100,000,000,000s, are still not quite… self-driving. The vast majority of robotic deployment is either pre-programmed or teleoperated.
Jensen Huang, CEO, Nvidia: “The ChatGPT moment for robotics is coming” Jan 2025